In this podcast, we are joined by Dr. Paul Croarkin of the Department of Psychiatry and Psychology at the Mayo Clinic Rochester, Minnesota, and Dr. Arjun Athreya of the Department of Molecular Pharmacology and Experimental Therapeutics at the same institution.
The focus of this podcast is on the JCPP paper ‘Evidence for machine learning guided early prediction of acute outcomes in the treatment of depressed children and adolescents with antidepressants’ (doi.org/10.1111/jcpp.13580).
Paul and Arjun set the scene by detailing what they looked at in this study, providing us with a summary of the paper, plus sharing insights into the methodology used for the research, before turning to the key findings.
In their paper, Paul and Arjun describe their work as representing a first step in establishing a symptom-based tool, and in this podcast, they detail what the next steps are, including how the tool could be used to measure a variety of other treatments. Paul and Arjun also comment on how this tool could be applied to extracting response trajectories to Cognitive Behavioural Therapy (CBT).
Paul and Arjun then turn to the translational opportunities for their research, including how they envisage their research being translated and what the implications of their findings are for CAMH professionals.
Subscribe to ACAMH mental health podcasts on your preferred streaming platform. Just search for ACAMH on; SoundCloud, Spotify, CastBox, Deezer, Google Podcasts, Podcastaddict, JioSaavn, Listen notes, Radio Public, and Radio.com (not available in the EU). Plus we are on Apple Podcasts visit the link or click on the icon, or scan the QR code.
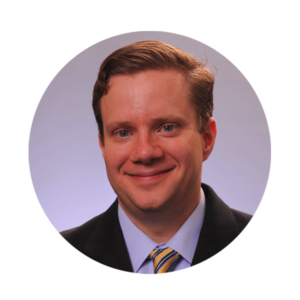
Dr. Croarkin is a Professor of Psychiatry and the Research Co-Chair for Child and Adolescent Psychiatry at the Mayo Clinic College of Medicine and Science. Dr. Croarkin’s research program focuses on adapting and innovating emerging technologies to advance the practice of child and adolescent psychiatry. This includes studies with wearables, artificial intelligence, and biomarker work to optimize diagnostic practices and the delivery of brain based interventions such as transcranial magnetic stimulation. A central theme focuses on the role of gamma-aminobutyric acid (GABA) and glutamate neurotransmitter systems in early-onset mood disorders with the goal of informing safer and more effective biologic treatments. This research is funded by a variety of industry, federal, and foundation grants.
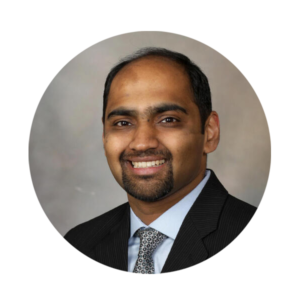
Arjun P. Athreya, Ph.D., M.S., develops novel methodologies and tools that embody innovations in artificial intelligence and machine learning that aid in translational research and clinical decision-making. His research combines patient data relating to genomics, metabolomics, imaging and electronic health records to identify biomarkers of disease biology (discovery science), drug response (clinical science) or both. Particularly, the technologies developed by Dr. Athreya are to be used as means of augmenting physicians’ decision-making abilities. These technologies aid in optimizing treatment selection and treatment management to ensure that patients get the treatment with the highest likelihood of delivering the therapeutic benefit with minimized chances of side effects. (Bio from Mayo Clinic)
Transcript
[00:00:32.062] Jo Carlowe: Hello. Welcome to the In Conversation podcast series for the Association for Child and Adolescent Mental Health, or ACAMH for short. I’m Jo Carlowe, a freelance journalist with a specialism in psychology. And I have with me Dr. Paul Croarkin of the Department of Psychiatry and Psychology at the Mayo Clinic Rochester, Minnesota; and Dr. Arjun Athreya of the Department of Molecular Pharmacology and Experimental Therapeutics at the same institution.
Paul and Arjun are co-authors of the paper ‘Evidence for machine learning guided early prediction of acute outcomes in the treatment of depressed children and adolescents with antidepressants’, recently published in the Journal of Child Psychology and Psychiatry. The JCPP is one of the three journals produced by the Association of Child and Adolescent Mental Health. ACAMH also produces JCPP Advances and the CAMH.
If you’re a fan of our In Conversation series, please subscribe on your preferred streaming platform. Let us know how we did with the rating or review, and do share with friends and colleagues. Paul and Arjun, welcome. Can you each start with a brief introduction?
[00:01:39.255] Dr. Paul Croarkin: Thank you for having us. My name is Paul Croarkin. I’m a professor of psychiatry at the Mayo Clinic in Rochester, Minnesota. My work is split pretty much 50/50 with a busy clinical practice across the continuum of inpatient intensive outpatient programming and outpatient practice. The other part of my time is involved in research that’s focused on novel diagnostic tools and therapeutics for child and adolescent psychiatry disorders.
[00:02:05.260] Jo Carlowe: Thank you. Arjun?
[00:02:06.640] Dr. Arjun Athreya: Hello. Thank you for having us as well. I’m Arjun. I’m a junior faculty in pharmacology. I’m [INAUDIBLE] computer engineering– computer engineer by training, and I spend a majority of my time developing predictive methodologies for drug response or biomarkers for rare disorders. I also invest a lot of time in teaching and training fellows and pre-doctoral students.
[00:02:31.760] Jo Carlowe: Thank you very much. Let’s turn to the paper, ‘Evidence for machine learning guided early prediction of acute outcomes in the treatment of depressed children and adolescents with antidepressants’, published in the JCPP. What did you look at in this study? Can you give us a summary of the paper?
[00:02:49.540] Dr. Paul Croarkin: This was an effort to look at, in my view, what is still a substantial clinical problem. We’ve come a long way as a field in developing evidence-based tools and guidelines for the treatment of major depressive disorder in children and adolescents, but an ongoing question, given the heterogeneity of childhood and adolescent depression, is what is the right type of depression and the right time to prescribe antidepressants? And there’s been many efforts on this.
And this seems like a deceptively simple problem, but it is not. Any clinician you talk to can vouch for that. The current effort was focused on using artificial intelligence tools with large existing data sets from landmark studies, clinical trials focused on fluoxetine, duloxetine, and placebo-treated patients. So, this involved using what’s called a training data set that my colleague, Dr. Athreya, will elaborate on further, to look at patients treated with major depressive disorder with fluoxetine, and then later on a testing data set in patients treated with duloxetine or placebo.
And the whole idea was to look at symptom trajectories from a depression screening instrument called the Children’s Depression Rating Scale Revised. And with this analytic tool and framework, Dr. Athreya was able to help us come up with a fairly accurate prediction for which patients will do well or not well with antidepressant medications, meaning response, remission, or failure to respond. The average accuracy for these various outcomes that we’ll talk about more is around 70%.
So, the idea is that this is an initial step that will, I think, move our field forward both on a psychiatric practice and more on — from a from a public health perspective as well.
[00:04:37.805] Jo Carlowe: Thank you. Can you us a bit more about the methodology that you use for the Research?
[00:04:42.740] Dr. Arjun Athreya: So, when we began collaborating together on this project, we both set out with the intention that we will build a predictive tool that is translatable to the average physician at the point of care. And that meant I had to seek his permission to observe their practice as an engineer and understand, how do physicians manage depression in routine care? And so, if I take a step back and broadly modularize their operations, a patient walks in, they get assessed for depression, and then a treatment is initiated.
They come back after four weeks, and now the physician has a judgment of not only how well the patient has responded or not, but they also know where the patient began prior to treatment. And then there is an adjustment of a dose if needed, or there’s a continuation of the medication. And then they come back after another four weeks, which is an 8 to 10-week period of the acute phase therapy. And then we know whether– if the patient is in remission– which is a near absence of symptom severity– at eight weeks, or is there a moderate response, but there’s still some lingering severity of depression– which we now call as a response in the manuscript.
Or did the symptoms not improved much at all, in which case, we would say it’s a non-response. So, if you look at it, there is a bit of a conditioning effect that these clinicians mentally work themselves out, which is, has the patient improved sufficiently from where they began? And then what happens between four and eight weeks? So, capturing that phenomenon, we developed what we call as probabilistic graph models that algorithmically capture that same logic that the physicians go through, and then use that paradigm to establish predictability of treatment outcomes at four weeks based on the severity of depression that the patient began at baseline and how much they have improved or not improved at four weeks, and then predict what happens at eight’ weeks. So that’s really where our methodological novelty comes into play.
[00:06:52.175] Jo Carlowe: And what did you find? Can you share some of the key findings?
[00:06:55.613] Dr. Arjun Athreya: We found that there are certain symptoms out of the 17 or so depressive symptoms that are comprising the CDRS revised rating scale, which is the Children’s Depression Rating Scale that is routinely assessed in the clinic, especially in research settings. And then we found that there are a set of these six symptoms that show very early demarcation of a change between those who are clear remitters at four weeks and will be sustained remitters at eight weeks or 10 weeks.
Or there are some groups of these symptoms where the improvement is — also sufficient enough for us to tell us that this is not a remitter, but neither a non-responder, but will be a good responder at 8 to 10 weeks. And the same symptoms also showed that the improvement is also insufficient at four weeks itself that we can say that this patient will be a non-responder at 8 to 10 or 10 to 12 weeks.
So we were able to find the threshold of improvement that is sufficient enough for us to prognosticate and predict what will be the outcome at the end of the acute phase. And I think that’s where the clinicians needed the input in terms of, hey, if at four weeks– do we know what this patient’s response will be at 8 to 12 weeks?
[00:08:14.650] Jo Carlowe: So, this really will be an important tool. And in the paper, you describe your work as representing a first step in establishing a symptom-based tool. What are the next steps?
[00:08:25.440] Dr. Paul Croarkin: In my view, there are many next steps in somewhat broad and different directions. We currently are collaborating on preliminary efforts to prospectively validate and translate this algorithm into practice. So, the idea is, could it be used in a large primary care environment as part of eventually, for example, an electronic health record tool that clinicians would have at the ready to not replace their judgment by any means, but uses a data point, much as a blood pressure or a lab test for other disorders.
There are also opportunities to study this type of approach for other interventions, which is something I’m very excited about– for example, novel treatments such as transcranial magnetic stimulation and other evolving treatments for depression.
Third, I think the story’s not over in terms of building and refining the algorithm. Ideally, in the future, these kind of tools would be set up to basically learn and improve with input. And we could individualize care not only patient to patient, but in treatment settings. So, for example, one large clinic or practice– the algorithms could adapt to what is found and what’s unique about the practice and the patients that are cared for in that clinic. And along with that, I think there’s much work to do in terms of the integration of other objective measures.
This is a first step with a very important rigorous measure of depressive symptoms. But we could look at other measures such as data from wearable technologies, lab measures, genomic measures, brain imaging, EEG. Dr. Athreya, have I covered everything, or do you have anything to add on this?
[00:10:07.770] Dr. Arjun Athreya: Yeah, I think, when we look at a lot of health treatment, one of the biggest issues that we see is, in the access to mental health care itself, there is a shortage of specialist care providers like Dr. Croarkin and his colleagues. And the question is, how do we use digital interventions and digital technologies to reach out to those who may not have access to a trained child psychiatrist even within 100 miles or kilometres of their home?
And this is really where future of work for the mental health providers and health care as a field– and see how we can better engage with patients, and monitor the symptoms, and provide in what we now call a decentralized fashion. So that’s really one of the many things that we are focusing on going next, which is to understand the ways in which digital health can become a reality for child and adolescent mental health.
[00:11:01.380] Jo Carlowe: You mentioned that the tool could be used to measure a variety of other treatments, and in the paper, you mentioned it could be applied to extracting response trajectories to CBT. Is that something you’re planning to look at?
[00:11:14.280] Dr. Paul Croarkin: We have ongoing efforts, nothing definitive to share at this point. But that is another exciting area.
[00:11:19.710] Jo Carlowe: Can you say any more, or too early?
[00:11:21.360] Dr. Paul Croarkin: It’s very early at this stage.
[00:11:23.235] Jo Carlowe: Let’s turn to the translational opportunities. How do you envisage your research being translated?
[00:11:29.158] Dr. Paul Croarkin: As we mentioned, the first thought would be that this, in a fairly short time frame, could be integrated– my first thought would be it would be ideal for what we call an integrated behavioural health environment. This is a large setting for large primary care clinic, or a — often a number of clinics, that engages mental health resources, such as this child and adolescent psychiatrist. But the child and adolescent psychiatrist has a very different role than what you would expect.
The role is to look at the treatment of youth with depression, psychiatric disorders at more of a population level– so not necessarily see every patient that comes through clinic, but develop rigorous evidence-based guidelines for paediatrician and family practice colleagues to diagnose and treat depression with things like CBT, and SSRIs, and SNRIs. So, the idea would be that, along with our ongoing IBH practice and the work that’s been done in that realm over the last decades, would be to incorporate a tool such as this to inform this practice.
So, this would be one more evidence-driven tool for a psychiatrist or a mental health professional in this role, as well as the primary care physicians that are served. So, to make it more tangible, these depression measures would be collected at baseline two weeks later, four weeks later, six weeks later, eight weeks later. And this may, as Dr. Athreya pointed out, won’t necessarily be done in the clinic.
It’ll be– in some settings, will be, with how things are evolving, remote measures. So, this current tool could be incorporated into an electronic health record, with the idea that entering these data would give the primary care colleague and the psychiatrist or psychologist working on the team early ideas about how patients are doing with certain treatments and what we’re looking at, again, as antidepressants.
It would speed up the decision-making process. It, I think, would be very helpful for treatment teams and very well-received by patients, because as you– I think you’re aware that we often do this trial-and-error approach, where– take this medication for two or three months, and if and when it doesn’t work, we’ll have to go back and try a different dose or different medication. This would make that process more efficient, and data driven. Dr. Athreya, do you have other aspects to mention on this?
[00:13:53.253] Dr. Arjun Athreya: The other translational efforts are to understand how to deliver these findings efficiently to the providers. The success of any of these technologies is to ensure that these kind of tools impose minimum effort on the clinicians and in terms of the click fatigue, and burnout, information overload, and such. So, there’s a lot of educational efforts underway and research efforts underway to understand how to present these findings to the providers so that they see this as a positive augmentation to their practice, and not necessarily an information overload that adds to the existing burnout crisis in the health care workforce.
So that’s something that we’re also very serious about in our ability to successfully translate the findings to the bedside.
[00:14:35.760] Jo Carlowe: Thank you. What else are the implications of your findings for CAMH professionals?
[00:14:40.957] Dr. Paul Croarkin: That’s a good question. We’ve talked about many of them already. But I think the take home message that I would like to convey along the lines of what Dr. Athreya was talking about– that we don’t envision this as a– ever as a replacement for human clinical judgment with things like cognitive behavioural therapy, anti-depressant prescriptions as well. This will simply be a tool, a data point to form our practice, again, much like other fields.
For example, the decision to prescribe medications for hyperlipidaemia– they have similar tools. Again, the blood pressure is another example. We need more objective data and tools to guide our practice to improve our outcomes, because inherently, if you look very carefully at what we do, we help a lot of children and families as a field, but there are numerous what I would call soft spots in what we do, because it’s highly, highly subjective– not always reliable in terms of our assessments and patterns.
Patients notice these things. It’s interesting talking to patients that have been in treatment over the years that we’ve visited numerous clinicians, and every physician or mental health provider says something different in terms of diagnosis and treatment plan.
[00:15:56.785] Dr. Arjun Athreya: Yeah. I just want to add to Dr. Croarkin’s thoughts here. Coming purely from academic perspective, we have to be cognizant of the fact that, very clearly, this is an augmentation tool. We are not replacing anybody’s judgments. Clinicians are still going to be the ones who are going to be the eventual decision makers in how they manage their patients, but there is that bidirectional interplay of opinions and thoughts from both the patients and the providers in terms of what these technologies mean to them.
So, there’s a very complex ethical conversation that can be had. And I think that’s really where we are investing a lot of our time, into understanding the bioethics of tools and technologies in the bedside. How are physicians perceiving it? What are their concerns– and also how they like these results to be presented to them, as I mentioned earlier the translational effort.
And I believe, if we truly understand these concerns and their inputs, and we understand how to deliver the results to them, we are moving closer to integrating these technologies in routine practice that physicians will then begin to interact with as part of their normal operations, as opposed to, hey, there is a tool like this– let me spend another five minutes figuring out what it does.
So, I think this is one of the many steps in that educational process. Hopefully the ongoing research efforts will help pave way to understand those constraints as well.
[00:17:25.300] Jo Carlowe: Have you detected a reticence amongst CAMH professionals about the use of machine learning, a sort of fear that it would take their place?
[00:17:34.652] Dr. Paul Croarkin: From my perspective, this is a great question. It’s been a global concern across specialties outside of mental health as well. And that’s part of the reason we’re highlighting this, because this is often misunderstood, what the purpose of this line of research is.
[00:17:52.197] Jo Carlowe: Are you planning any follow-up research that you can share with us?
[00:17:55.790] Dr. Paul Croarkin: We’re in the very early stages of prospectively validating this tool, and we also hope to integrate biomarkers into similar analytic frameworks. And also, we will be looking at other interventions, as you mentioned– cognitive behavioural therapy outcomes as well as a novel treatments for depression.
[00:18:17.355] Jo Carlowe: Is there anything else in the pipeline for either of you that you’d like to mention?
[00:18:22.450] Dr. Arjun Athreya: Well, we have begun some of these next steps already in terms of digital health interventions. And these are all pilot studies that we are conducting. Terms of understanding, what are the challenges of even remote management of mental health or mental health disorders– so what does it even entail from an engineering perspective, both in terms of the quality, the fidelity, and noise, and things like that? And also, how do we engage with our patients?
So I guess this is something that we have embarked on a small scale, but we are in very early days. And hopefully, in the years to come, we would have understood, what does it really take to put these technologies into the hands of the patients and the providers, both from an intervention perspective, but also from a monitoring perspective? So, I guess this is an exciting future for us, and it is critical that we have these close partnerships between engineers, and clinicians, and bioethicists, and life science researchers as well.
[00:19:23.500] Dr. Paul Croarkin: One more specific detail that is well underway– another colleague that’s a co-author on the manuscript, Dr. Magdalena Romanowicz along with Dr. Athreya are currently spearheading a very exciting studies really, with the idea of looking at parent-child interaction therapy, an evidence-based form of therapy for young children with disruptive behaviours, and often aggression. And the idea is to use modern tools, data from wearables, and analytic tools that we’ve been talking about today to inform the application of this therapy that’s already highly effective.
But we hope that these efforts will make this even more individualized and provide– as Dr. Athreya alluded to provide opportunities for greater access through decentralized remote applications of these forms of behavioural therapy.
[00:20:18.500] Jo Carlowe: It all sounds very exciting, with great potential. Finally, what take-home message do you have for those listening to our conversation?
[00:20:26.610] Dr. Paul Croarkin: We’ve enjoyed speaking with you this morning, and we’re excited about this early preliminary work that’s focused on using a evidence-based artificial intelligence approach that we think will guide and assist with decision making for the application of treatments. Hopefully we’ll be able to talk to you again, Jo, in two or three years about prospective studies and some of the other efforts we’ve talked about as well.
[00:20:53.170] Jo Carlowe: Thank you both ever so much. For more details on Dr. Paul Croarkin and Dr. Arjun Athreya, please visit the ACAMH website, www.acamh.org, and Twitter @acamh. ACAMH is spelled A-C-A-M-H. And don’t forget to follow us on your preferred streaming platform. Let us know if you enjoyed the podcast with a rating or review, and do you share with friends and colleagues.