In this Papers Podcast, Isaac Ahuvia discusses his JCPP paper ‘Evaluating a treatment selection approach for online single-session interventions for adolescent depression’ (https://doi.org/10.1111/jcpp.13822). Isaac is the lead author of the paper.
There is an overview of the paper, methodology, key findings, and implications for practice.
Discussion points include:
- Definition of single-session interventions.
- How the treatment selection algorithms were created and tested.
- Implications for future research and front-line clinicians.
- Will these types of machine-learning algorithms be refined to be usable for the future?
In this series, we speak to authors of papers published in one of ACAMH’s three journals. These are The Journal of Child Psychology and Psychiatry (JCPP); The Child and Adolescent Mental Health (CAMH) journal; and JCPP Advances.
Subscribe to ACAMH mental health podcasts on your preferred streaming platform. Just search for ACAMH on; SoundCloud, Spotify, CastBox, Deezer, Google Podcasts, Podcastaddict, JioSaavn, Listen notes, Radio Public, and Radio.com (not available in the EU). Plus we are on Apple Podcasts visit the link or click on the icon, or scan the QR code.
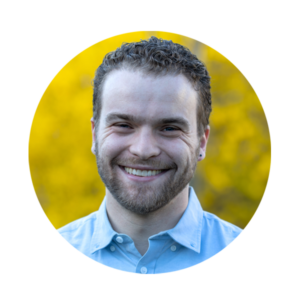
Isaac Ahuvia (he/him) is a Ph.D. candidate in the Clinical Psychology program at Stony Brook University. His research focuses on the ways that adolescents understand depression, and how their beliefs about depression shape their experiences and clinical outcomes. As a member of the Lab for Scalable Mental Health, he has conducted research on treatment matching for single-session interventions, depression belief change through single-session interventions, and has co-designed a single-session intervention targeting body dissatisfaction.
Transcript
[00:00:01.310] Mark Tebbs: Hello, and welcome to the Papers Podcast series for the Association for Child and Adolescent Mental Health, or ACAMH for short. I’m Mark Tebbs, and I’m a Freelance Consultant. Today, I’m really pleased to be talking with Isaac Ahuvia, who’s the Lead Author of a paper entitled “Evaluating a Treatment Selection Approach for Online Single-Session Interventions for Adolescent Depression,” recently published in the Journal of Child Psychology and Psychiatry. Isaac, thank you for joining me. Really looking forward to our conversation today.
[00:00:37.870] Isaac Ahuvia: Thank you so much for having me.
[00:00:39.550] Mark Tebbs: Good stuff. So, could we start just by you introducing yourself and the people that you worked with on the paper?
[00:00:47.420] Isaac Ahuvia: Yeah, so, I’m a PhD candidate at Stony Brook University in New York. The study was led by members of the Lab for Scalable Mental Health, which is Jessica Schleider’s lab. She’s on the paper, along with Michael Mullarkey and Jenna Sung, and we also worked with Kathryn Fox at the University of Denver.
[00:01:06.270] Mark Tebbs: Excellent, thank you. So, could you just give us a little bit of a brief overview of the paper?
[00:01:12.840] Isaac Ahuvia: Sure. So, this study was using data from a randomised controlled trial of two single-session interventions and the question here was, you know, we know that both interventions are effective on average, but can we find a way to match people to their, sort of, optimal intervention, and in doing so, hopefully, increase the effects that these interventions have?
So, this was a large online study with about 1,000 adolescent participants. We developed algorithm to try to match people to what we thought would be their optimal intervention and then, we evaluate that by comparing how they actually did to how we think they should’ve done. And ultimately, what we found was that it was actually quite challenging to tell how well somebody would do with one intervention than with the other, and we were not really able to match people very effectively. We’ll get more into why exactly we think that is.
[00:02:12.989] Mark Tebbs: Thank you. That’s a great introduction. So, could you tell us a little bit more about, maybe, your original research objectives? It’d be interesting to know, kind of, why you wanted to study this area, and maybe just to, kind of, define some of those terms. So, you know, it’s, kind of, about online single-session interventions. So, if you could tell us a little bit more about what they are, that would be really helpful.
[00:02:37.540] Isaac Ahuvia: Yeah, absolutely. So, when we say single-session intervention, we’re talking about interventions, in this case, both of these are for depression, but we mean any kind of psychological intervention that is intentionally designed to only last for one session. So, what we know about long-term psychotherapy and other long-term interventions is that quite often, those turn into just one session, because somebody will just engage for one session, then they won’t come back. Dropout rates are very high, they’re very hard for people to access, and so, the, sort of, mindset that we’re bringing into this work is if we can only interact with somebody for even a few minutes, if that’s the reality of it, is there a way that we can, sort of, distil the main ingredients of the intervention into something that we can give someone in that time, such that they can still see some positive benefits from that?
And in the case of the two interventions we look at here, we find that that is the case. So, this particular study is building off of a randomised controlled trial that we already did with these two interventions. The two interventions are both online single-session interventions. Project Personality is trying to instil in depressed adolescents a growth mindset about personality, a sense that their personality, their emotions, their experience, can change, and try to give them more of a sense of hope in that way. And the Action Brings Change Project, which is the second intervention, is a behavioural activation intervention which is trying to teach adolescents that when they’re feeling down and depressed, they can do things to try to change their mood.
So, both of those have positive effects for the participants, even in just the single-session, but because they are really, at least on their face, quite different in the mechanisms of how they work, we were trying to see, well, are there some adolescents who are going to respond better to one than the other? And if there are, can we match those people to the intervention that’s going to work best for them?
[00:04:39.530] Mark Tebbs: So, let’s turn to the methodology. How did you go about creating and testing these algorithms, and were there any particular challenges that you had to overcome?
[00:04:50.960] Isaac Ahuvia: Yeah, sure. So, the data that we have is, again, it’s from a randomised controlled trial. So, we have about 1,000 adolescents and half of them did the first intervention, half of them did the second. And so, we can see from that how well they actually responded in reality to the intervention they were assigned to. Of course, the challenge is we can’t tell how well they would respond to the one that they were assigned to, and so, without that information, the way that you have to go about a study like this is you have to start by predicting a response to each of these interventions. And so, that’s the first step, trying to build a predictive model that is going to predict for each participant how well they would respond to, in this case, Project Personality, and then, how well they would respond to the Action Brings Change Project.
Once you have those predictions, you can determine what is each person’s optimal intervention, at least as far as, as you can tell with your predictions. So, if you imagine, let’s say, a hypothetical girl, Ella, let’s say she’s 14-years-old, you know, we know her gender, we know her age, we know something about her symptoms. Maybe she’s experiencing more mood symptoms than somatic symptoms. Maybe she is feeling really hopeless. So, we have all this information and we’re using this information to say okay, how well do we think she would respond to Project Personality and how well do we think she would respond to the Action Brings Change Project? And let’s say we predict that she would respond very well to Project Personality and not very well to Action Brings Change. Now, we can say, okay, for Ella, her optimal treatment is Project Personality. That’s the second step.
Now, the way that we evaluate this kind of algorithm is by comparing people who, in reality, were assigned to the intervention that is their optimal treatment and those who were assigned to the other one. So, we have – for all 996 participants, we know what we – how we think they would respond to each intervention. We know what we think their optimal intervention is, and if our methods for doing that are effective, then what we would expect is people who are assigned to their optimal intervention will do better than people who are assigned to their sub-optimal intervention.
[00:07:05.880] Mark Tebbs: Well explained. That was quite a tricky subject and you have explained that really well. So, what did you find?
[00:07:11.849] Isaac Ahuvia: So, what we found was, kind of, surprising, which is we really didn’t find that people who were assigned to their optimal intervention responded all that much better than people who were assigned to their sub-optimal intervention. Now, again, both of these interventions, we already know they’re effective, but for people who are – you know, we call them lucky, because they are randomly assigned to the intervention that, in retrospect, we think would be best for them, they improved by about two thirds of a standard deviation on their depression symptoms, from before they took the intervention to three months afterwards. And then, for the people who we think are unlucky, they also responded by improving about two thirds better in terms of standard deviations. A slight amount less, but not in a statistically significantly difference.
And so, that was really quite surprising to us and in trying to figure out why that is, we started to look closer at the predictions that we were making. That’s the foundation of this whole comparison, the predictions we’re making about how well someone’s going to respond to one or the other intervention, and we saw a couple of things. So, first of all, the predictions we’re making about how well each participant is going to respond to each intervention, ultimately, were not that powerful. So, when we predicted people, how well they would respond to Project Personality, compared that to how well those people actually did respond, for those who were assigned to that, correlation was, kind of, weak. It was about .4. And when we did the same for the Action Brings Change Project, the correlation is about .25. So, in other words, when we’re making these predictions, our predictions are only explaining, really, about 10% of how well someone’s actually responding to the intervention. So, that is already, sort of, hamstringing us here.
And then, moreover, when we look at how well somebody was predicted to do to the Project Personality versus to the Action Brings Change Project, those predictions were actually really similar. So, if you think back to, you know, this hypothetical adolescent, Ella, what we’d really like to see for this kind of, you know, treatment selection algorithm to be effective, is for the algorithm to say, okay, Ella would do really well with Project Personality and not so well with the Action Brings Change Project. But what we ended up finding was the participants who were supposed to do well with one intervention were also supposed to do well with the other one, and those that were not supposed to do well with one also weren’t supposed to do well with the other. Those predictions were really highly correlated, at about .8.
So, big picture wise, the predictions we’re making about how people are responding to these interventions are not super accurate to begin with and they’re also not doing a great job of distinguishing one intervention from the other intervention. And ultimately, that’s what we ended up seeing the results that we did, which is that the treatment selection algorithm was really not making a big difference.
[00:10:02.490] Mark Tebbs: Okay, thank you. So, are there implications of this from a future research perspective?
[00:10:08.650] Isaac Ahuvia: Yeah. So, our results really, kind of, echo what a lot of other studies are finding when they’re trying to do treatment selection in this way, which is, you know, sometimes there are significant effects of these algorithms, sometimes they are helpful. Usually, the effects are, kind of, small, and quite often there are not effects, which is what we found. So, implications wise, I mean, my biggest takeaway here is that it’s still really, really hard to predict how well people are going to respond to psychological interventions. In our study, we can just say that with regards to these two single-session interventions, but that seems to be really true with a lot of interventions, and it’s really hard to tell how well someone’s going to respond to one versus another. Both of those things, again, I think are consistent with at least some other research that has tried to do this with other interventions.
[00:11:04.760] Mark Tebbs: Do you think that the algorithms will be refined to a point where it is useful in the future?
[00:11:13.130] Isaac Ahuvia: That’s a good question. You know, the way I think about it is Clinicians make these kinds of decisions all the time, right? You have to decide if I have somebody who comes in, I do my intake, would it be better for me to use, you know, exposure methods with this person or some other kind of method? And we all have our own, kind of, algorithm that we’re using in our head to make these decisions. We also have really rich data, in the form of the case conceptualisation, from our interviews with clients.
So, when you look at this through more computerised data-driven version of this, it’s true that right now, first of all, the data that we’re putting into it is, you know, self-report data on symptoms and on, like I said, other variables, hopelessness, perceived control, things like that. The data might not be quite as rich as you might get from an actual interview with somebody. And then, the predictions, in this case, you know, we’re testing out a bunch of different machine learning models or seeing what works best, even though those are mathematically quite sophisticated, they might not be, you know, really quite so effective at this kind of prediction, right? Who knows?
So, I think as the technology improves, I would definitely expect at least some kind of improvement in these kinds of algorithms and how they’re doing for treatment selection. But for me, the question is always going to be if you compare it to somebody who is actually sitting down and doing, you know, a clinical interview with somebody and getting a really rich sense of how these symptoms interact and what, you know, what are actually the causal factors for somebody, compared to that, will the machine learning algorithm ever be able to, you know, replicate that at a large scale in this data-driven way? And I don’t know, it doesn’t really feel that way to me right now, but who knows?
[00:13:10.120] Mark Tebbs: Yeah, the jury’s out. So, from a practitioner perspective, and I’m thinking for a Clinician, kind of, working in the field now, so are there implications or, sort of, takeaway messages for, kind of, frontline Clinicians?
[00:13:23.560] Isaac Ahuvia: Yeah, so, like I said, I think we all, kind of, do this kind of work. You know, and we’ll have our own, kind of, algorithms that we’re using. The implications of this research for Clinicians, I think, is, you know, if we’ve found that we could use a machine learning algorithm and, you know, just some questionnaire data from potential clients and that we could really easily tell how well somebody would respond to one intervention versus the other, then I would say, you know, be on the lookout for tools like this. They could really help your practice, you know, help you make those kinds of decisions in more types of data-driven ways.
I don’t know that we’re quite there yet, but I do think in another way, these kind of results are a good reason to, kind of, reflect on how Clinicians, you know, how you are making these kinds of decisions, right? This is one way to make these kinds of decisions in a very, again, data-driven way using these, you know, empirical validated measures of symptoms and whatever else. And as Clinicians, I think we have to ask ourselves, when we’re doing this kind of work and making these kinds of decisions, are there ways that we can do that better? That might not right now include, you know, using some kind of machine learning algorithm, like, bringing our clients’ data into it and so on, but I think there are always ways that we can make those kinds of decisions better.
[00:14:48.000] Mark Tebbs: Brilliant, thank you. So, are you planning any follow-up research? Is there anything, kind of, in the pipeline that you’re able to share with us?
[00:14:57.329] Isaac Ahuvia: Yeah, absolutely. So, in the Lab for Scalable Mental Health, we’re always doing more research on single-session interventions. In the case of these two interventions, so Project Personality and the Action Brings Change Project, we already have good evidence that they’re effective. And so, the focus right now is on, first of all, actually disseminating these interventions and making sure people can access them as easily as possible, and research wise, trying to get a better understanding of what the mechanisms are.
One, kind of, takeaway that you could have from this particular study is well, even though these two interventions appear to be targeting very different things, they actually might have, kind of, similar mechanisms. That would be consistent with the finding that when people do well with one, we think they also do well with the other. But we need more research to try to figure that out. So, that’s something that we’re working on. We’re also working on other single-session interventions. I’m working with Arielle Smith, who’s a brilliant Project Co-ordinator in our lab, on the intervention targeting body dissatisfaction in adolescents, called “Project Body Neutrality.” We have a pilot study of that published and we’re working on the RCT, as well.
And then, I should also say that all of these interventions that we have are publicly available and you can get them all on our website, at schleiderlab.org/yes. Schleider is spelled S-c-h-l-e-i-d-e-r lab.org/yes, and that’s, kind of, the repository for all of these interventions.
[00:16:28.920] Mark Tebbs: Isaac, it’s been really lovely speaking to you. Is there a, kind of, final message for our listeners?
[00:16:35.500] Isaac Ahuvia: I mean, for me, the takeaway from this research is that it is just still really hard to predict how exactly people are going to respond to different psychological interventions. That’s really been the take home lesson for me, and I hope we can continue to get better at it, but for now, it’s really a challenge.
[00:16:54.870] Mark Tebbs: Thank you so much. It’s been a really interesting, kind of, conversation, but for more details on Isaac Ahuvia, please visit the ACAMH website, www.acamh.org, and Twitter @acamh. ACAMH is spelt A-C-A-M-H, and don’t forget to follow us on your preferred streaming platform, let us know if you enjoy the podcast, with a rating or review, and do share with friends and colleagues.