The prevalence of suicide and self-harm in children and adolescence is a subject we should all be concerned with. For the 2019 Judy Dunn National Conference we gathered together the leading academics, clinicians, and researchers in fields of self-harm and suicide, plus those who have lived experience.
The lecture here is from Prof. Paramala J Santosh ‘Mobile applications and wearable devices in assessing suicide risk’. Paramala is a Consultant Child and Adolescent Psychiatrist, developed and heads the Centre for Interventional Paediatric Psychopharmacology and Rare Diseases.
ACAMH members can now receive a CPD certificate for watching this recorded lecture. Simply email membership@acamh.org with the day and time you watch it, so we can check the analytics, and we’ll email you your certificate.
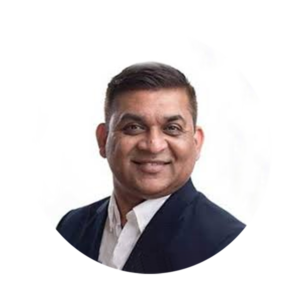
Paramala is a Consultant Child and Adolescent Psychiatrist who developed and heads the Centre for Interventional Paediatric Psychopharmacology and Rare Diseases (CIPPRD) at the Maudsley Hospital, London. A Visiting Reader at the Institute of Psychiatry, Psychology, and Neurosciences (IoPPN), King’s College London, he focuses on translational research. An international expert on Autism, developmental psychopharmacology, neuropsychiatry, paediatric neurodegeneration, suicidality, and the use of information technology to improve health delivery. His research includes the overlap of Autism, ADHD, and Bipolar Disorder; psychopharmacology and paediatric neurodegeneration in the context of Hunter syndrome, Hurler syndrome, Sanfillipo disease, Gaucher disease, Neiman-Pick Type C, Rett syndrome, and other diseases. He is recognised expert in the field of assessment and management of complex multiple co-occurring developmental disorders. Currently, Paramala is involved in research of comorbidity in Autism Spectrum Disorders, its assessment and management, as well as the MILESTONE project which looks at how to improve transition process and experience for young people from Child and Adolescent Mental Health Services to Adult Mental Health Services, in the UK and across Europe. Added to this he has also been involved in developing computerized assessment tools such as the HealthTracker™.
Transcript
Thank you. I just want to say I need something in terms of timing, and because I’m very bad at that [laughter]. I’m not at all like previous speakers, so I apologise in advance. What I’m going to say because I was not here to listen to the first two speakers I’m really sorry if I do something and you classify it as not really PC in this particular scenario. All I’m wanting to say that my expertise doesn’t specifically lie in suicidality or self-injury. I came into this field by chance, primarily because my expertise lies in pharmacology and I see children with all sorts of conditions which are treatment resistant. And so in early 2010, there was a call and that was the time when SSRIs and anti-epileptics and stimulants and everything were being accused of being creating suicidality in children. So I won a three million grant from the EU to coordinate and run a project called Suicidality: Treatment Occurring in Paediatrics, which is to see what is the relationship between medication, prescription and suicidality. So we realised at that time that there was hardly any instrument that had been identified, that had been specifically built for that purpose because when we look at the Columbia scale that was completely for a different reason. That was never intended to be used as a screening instrument at all, so therefore our inclination became that we had to first create new instruments; it has to be online, so that young people can use it; it has to be tested in medical environment, in psychiatric environment, those who are depressed, those who are not depressed and in the general population. So that was the mission that we were [inaudible 00:02:16] and then to look and see whether any medicines increased suicidality.
So that was what the project was about. So that’s how I got into this field and I will take you to what happened since. And so in terms of the competing interest I am the director of the company called HealthTracker, which is the one which provides the web-based platform. This is a platform which I developed along with Paul Gringras, who is a professor of sleep medicine, whilst I was working at Guys and St Thomas’s and Great Ormond Street, so the IP partly belongs to the NHS. They get royalties back from using the system. I was the coordinator of the project, an ECAP project called STOP, and I received funding from a few companies who deal with molecules for rare diseases because I run the Rare Disease Centre and I don’t have access to these molecules unless I’m working with the company. And so that’s the… and I work with patient organisations as well.
So this is what we do in terms of where any patient comes into my team for health what we do is that we actually have an integrated model that’s patient centred care. We use a biopsychosocial approach. We get all of the information that is needed. We collect physiological data using a device called [inaudible 00:03:42] at the moment. We’ve tried various devices and we’re probably going on to checking out others as well. And what this does is that it collects information on heart rate variability, [inaudible 00:03:50] data, temperature changes, and so on. And what we do is that on the Health Tracker we’ve got a whole host of instruments. Depending on the kind of patients we see, it automatically allocates the questionnaires that are necessary, so the patient can do it, the parent can do it, the teacher can do it, the clinician can do it, and it automatically scores and you get everything onto the screen in one pick. You get all information, multi-source information on a single screen. So that as a clinician it becomes a lot easier for you to have the single screen in front of you, get all the information together, and then you can set the scene for what happens.And so, for example, we have medication side effects, you have your psychotic symptoms, you have treatment response, how they respond to treatment, quality of life, burden of families, suicidality, for example, the stock set of questionnaires are there, allocated according to a four question screener. If they score positive on any of the four questions then the rest of them are allocated. So that’s the background to why I’m here. This is a complex dive into… which I pinched from another article. Only we’re talking about is when you’re looking at suicidality in the broadest sense, whatever we call it, whether it is self-injury, whether it is self-harm, you have multiple drivers. And unless you’ve got the mechanism by which you can actually collect information on all of these, you do not know what drives the process for whom because it is completely illogical to think that you have one model for everyone. It does not work like that in medicine. And human beings are different. Different drivers exist for different people. We need to personalise it if you want action to be relevant to them.
So that’s really what the… So part of it is… This has only come out recently, but you can stop now when I look back on mapping, what we’ve identified as drivers and we’ve actually gathered instruments for almost every single thing in here has been collected as information from the project. So if you’re looking at it just in terms of suicide rates, it’s not surprising 15 to 49 year olds are the most [inaudible 00:06:09]. Now, I think that as, even in the last ten years, things have moved on in terms of if you’re looking at completed suicides. So, for example, we now know that if a person has high functioning autism they are eight to nine fold likely to complete suicide. So, we’re not talking about attempts, we’re talking about completed suicides. And I’m talking about having to investigate… As a clinician, I’m asked to investigate when someone attempts suicide, completes suicide within an inpatient setting.
Now, nine times out of ten a completed suicide in an inpatient setting arises in the context of someone who’s got high functioning autism, either diagnosed, not diagnosed or treat significant enough, because they seem to have this very deliberate mechanism of being able to camouflage the issues. They are planned meticulously and they seem to have a method, a mechanism by which hopelessness seem to be dark as such; it’s black and white. So trying to get hope into those individuals is far greater than in the typical neurotypical population. So therefore… and this is all of the things that we’ve only realised very recently, so the one message I would say is that if you are looking at patients in your clinic, please don’t miss suicidality in high functioning autistic individuals who may smile and tell you in front of you that things are okay. And often if you look at the data they have told clinicians that they were suicidal. It’s the clinicians who have not taken it seriously because the person in front of them has had an inappropriate affect, because they’re smiling, they’re giggling, and that is because of the autism.
And so if you are looking at risk evaluation, using the glasses of the neurotypical patient, you will miss it completely because the risk, what you need to be looking at is ignoring what the patient looks like, listen to what the person is saying, take the family or clinician’s view or siblings’ view of what they think that the person’s mood is, and then put it all together and you get a risk evaluation, which is far more appropriate. And this is because if you look at the statistics, the number of people who are completing suicides is far greater than it should be in this population. And that’s why I’m studying this field, not for anything else to do the topic because I think it’s something that is very important to consider.
So if you look at the whole stress-diathesis model and this is what in 2010 when we were developing the thing we looked at. So we looked at all of these various factors. We made sure that we were collecting information on all of that. And when you look at suicidality very specifically, there is an issue if you’re considering the chemical, which is a… or a molecule that is actually somehow making it more problematic, whether it is a [inaudible 00:09:10], whether it’s an SSRI, whether it is Roaccutane; it could be any of them. So the issue with that is the model, which I’m describing now is that when you start the treatment initially what happens is that the agitation might increase, and this is why anyone who remembers when the first time SSRIs came into the market with fluoxetine, and I remember vividly the first month we had to prescribe benzodiazepine. That’s gone, forgotten. And that it how it was marketed right at the beginning for us, but now we don’t do that for very different reasons and good reasons, probably. But the issue is that there may be an agitation that increases initially, and then after that, by week two, three, it might come down and actually decrease, so net effect is good.
But if you’re looking at this line here, which has to do with suicide risk, you may actually have a fear that there is an increase in suicidal risk associated, so in terms of monitoring you need to do it differently. But what we don’t know is whether all the drugs have the same profile. This is for SSRIs. We don’t have a clue whether anti-epileptics have this, whether antipsychotics have this, or students have this. They may have a completely different take on how it is. So what we ended up doing was we had to develop an instrument that could be used universally for any drug in the market to try and see how you can actually do that, and, of course, the issue is that the mood improves much later than the energy improving. So when you have energy improvement, you can actually act on your thoughts.
Now, that said, if you’re looking at it from the perspective of technologies at the moment, you’re looking at very different things. And I have not gone into the details of trying to show you what works and what doesn’t work because I looked at it and decided I was not the right person to do it because most of them have never been tested in a method which needs to be done for mobile technology because you have to do certain steps and none of them have gone through it, so these are things… the better methods are there; the mobile methods are there, social networks and so on. Most of them have some evidence. Most of the people who are driving it are… If they stuck to the guidelines that are necessary for an approval of a therapy or a treatment they would not meet them. So that’s the problem at the moment. It needs to be tested further.
Now, when you’re looking at it from the perspective of suicide prevention aspects of it, you’ve got various things down here. And in this again, most of it what happens is to do with crisis management. Anything to do with crisis management it doesn’t matter how it’s delivered. It could be through the internet. It could be through [inaudible 00:12:00] identify, and so on. It is common sense. Crisis management help is… whether you’re looking at the future or what do you do it seems to have had an impact in terms of [inaudible 00:12:10]. And so if you’re looking at it, accessing peer support and safety plans are the most common aspects that seem to be relevant when it comes to using it. So if you’re looking for an app, if you’re looking for something, these other aspects of it, can you actually manage a good enough safety plan and peer support mechanism within that context of it? And is it something that does not breech the GDPR guidelines and actually has confidentiality associated with it? So those are high levels of scrutiny, and that’s part of the reason why you do not have anything that has been given the okay for it because they have not reached that level of things yet. So that’s all I’m going to be talking about in terms of the literature of that because unfortunately we do not have very much that I can talk to you confidently about.
So the study, which we… was using this was we had six countries involved, so we had all the countries listed there. And the overview of the project was that we had one which was to do with developing a questionnaire which has to do with risk factors and protective factors and medication related factors and suicidality assessment, So we have developed four different scales, we had to validate them, and then it had to be on a web-based platform, which had to be valid. Then we had to use the scales in seven different cohorts so that we could say that the scales could be used in medically unwell groups, psychiatrically unwell groups and in the healthy population. We then had to do machine learning with them. And in these other things, this is as important, which I’m not going to be talking about here, but one of the things that we did was we identified… we looked at the largest databases available in the world, looking at [inaudible 00:14:09] databases, the WHO databases and [inaudible 00:14:11] and everything. And we identified, based on the reports, how many of them had reported suicidality with a particular medicine, so we could identify the medication associated in children of different age groups. And surprisingly, there are many medicines that we use which actually reach the threshold for that. And then we used that information and incorporated it into the questionnaires as well. And this was a work package which identified how can you get good biological samples from people who self-injure or self-harm, and we published on that, so you can get saliva samples with just a cheek swab to get good genetic data from them, but surprisingly we found that if you are under eight years old, you need to double the amount of saliva because the shedding being different in terms of cheek cells. So therefore these are things that are also there that we put into the… and of course the training dissemination as well.
So we had 1,002 subjects in total and we had seven cohorts. Broadly speaking, these were individuals who were depressed. Half of them were on fluoxetine, half of them were on CBT, and this was because at that time we needed to identify whether fluoxetine made things worse and so on. But it was never doesn’t part of to answer that question. This was to develop the instrument, the platform for it. Then we had psychiatrically unwell but not depressed relevant, so most of them were neuro-developmentally unwell. Half of them were on aripiprazole, the other on risperidone. And then we had asthma and respiratory illness. Half of them were on montelukast. Montelukast was at that time, even now, it’s got a black box label for increasing suicidiality, so that’s because of its action on the interleukins as such. And then the others were non-montelukast peer treated. And then lastly, the general population, we collected 222 subjects at the same time.
One time to remember in suicide research is that if you connect normative data at different time point as such, societal changes may be different, like we’ve been just discussing about the NSSI. There could be a difference in terms of the rates. There could be difference of things. So we have to do it contemporaneously, so we could actually say that it was the difference on and on, so that’s what that was about. So these were all the things, the information, that we collected online, using the Health Tracker platform, as such, that we had a suicidiality assessment scale that assessed the acts, the ideation, the behaviour, the planning and how they aborted it, all of that, the risk factors, all the risk factors that were the known, and then resilience, what were the things that prevented them from acting on it, and then medication side effects. And this is the first time that there’s been a very specific scale developed to look out for side effects that increased suicidality very specifically.
So those were the thing and apart from that [inaudible 00:17:20] we have looked at the Silverman, the Columbia and then we had all of these, depending on the patients who were patients we had, and then we had an alert system available on the system, so that if scores increased too much you could get an automated… the clinician could get an automated email to alert… to tell them this person’s scores have gone up. And simply because for the project we could not activate that alert because the Ethics Committee said, by definition, if you did that, you would change practice and so therefore we didn’t. But we developed the whole process and it’s all ready to… and it’s being used in clinical settings at the moment. And then lastly, after all of that, we then used the data to use in machine learning in AI to see whether we can predict who attempted.
So the process of how we developed the scales, each of the scales as such… This is the MDA approved protocol, so that any instrument that follows this thing can be used in clinical trials. So what we do is you do a systematic literature review, identify the items in scales develop, and then you can have focus groups of patients, families, clinicians, everything, then you want to try the scales, then you get excellent feedback, and we had an experts advisory board. And then after that it’s uploaded onto the platform, and then the patients have to approve the look on the screen because otherwise if they’re not going to use it the data is useless. So everything had to be… and they give feedback. And then the final product is what then is tested, and for test we test 80 percent of subjects, we ended up between 87 and 100 subjects. We did it within a week to check, a week to two weeks to see whether the thing would change or not, and then for stable. And then the rest of it, we had more than 1,000 subjects in which we tested the whole model this year. And then finally we did the… so we had exploratory factor analysis and [inaudible 00:19:20] factor analysis and so on. Initially [inaudible 00:19:23].
So this is how the questionnaires look for individuals. This is just an example of how it is very easy to just click and go, so they could do it on their phone; they could do it on their iPad, whatever. So when you look at completions, that’s the issue when it comes to trials. You can have the best system in the world, if it is not completed, you can get wrong outputs from it as well. So the thing is that if you are looking at it, we have from baseline to one year, so we’re talking about 52 weeks that we had, and at the end point we had around 72 percent of people completing, so it was not too bad as such. And it was all of the scales. So the process was that you had a full item screener. If you were positive, you’ve got the whole battery. If you were negative, then you skipped some of them and then you did certain other scales which had a shorter version of it because we had… before we got to this, we identified that when everyone did everything, if you missed… if you had a zero for all four of the screening questions there was less than one percent who scored even on one item on the rest of the questionnaires. So therefore, it was pointless doing the whole thing. And so that’s what we ended up using as part of the decision making model. And then you could use it to… what we have is that we’re using the [inaudible 00:20:48] assessment scale, we could then impute what would be the score on the Columbia rating scales because the mathematical modelling was done at the back end, so that we could compare if you use the STOP scale it would still compare what a Columbia rating scale would have scored if someone had done it. So that was also done. And then we could get the nomenclature either by Silverman or the [inaudible 00:21:12].
So this is an example of the scale. So what happened was that we had a whole lot of suicidal ideation here. You had the suicidal behaviour and then you had plans and things. So these were the three factors that came up in the suicidal assessment scale. Now, when it comes to side effects as such, we had a whole lot of items to do with medication [inaudible 00:21:35] emotional dysregulation, psychotic or bipolar symptoms, and behavioural dysregulation. So these were the ones which came out from the whole thing. When it came to resilience factors you had external and internal factors, and as you can see you have internal locus of control and extent locus of control. And then when it came to the risk factors scale, you had a whole lot of risk factors which are not different from what you would expect, but these are to do with relationships, psychosocial factors, stressful experiences, substance misuse, chronic pain, all of that. Now, the important thing is that these were all scales that was done at different time points, so that patients would need to collect the information. So this is just to show you how it would have appeared to them, depending on the four scales, depending on what the patient had.
So what did we find when we collected all of this data? The first thing is that when you’re looking at it, suicidal ideation [inaudible 00:22:40] by adolescents, it did not matter which cohort you were looking at. All of them reduced in six months and by 12 months it was much lower than the baseline. It didn’t matter what you did. The treatment order in these seven cohorts did not produce a shift. When it comes to the ideations, suicidal ideation when it is done by parents, is rated by parents, exactly the same thing, by clinicians, exactly the same thing. So in other words, suicidal ideation as such, focussing on suicidal ideation as your main thing probably will be useful in short term, but it really doesn’t seem to be the main driver for what happens in terms of terrible outcomes. So overall suicidal ideation decreased in whichever cohort we looked at. So the final product from this STOP study is to actually have a profile of a patient like this, so you know this is the level of suicidal ideation, and so it shows green to red, and it tells you where it is and where the… So if you’ve got someone whose attempts has been in terms of [inaudible 00:23:49], then you know that you may need to do it. So it’s rather than it just being saying that someone is having an NSSI or self-harm or whatever, you actually have a whole profile of the person, so that you can plan what needs to happen.
Now, we had, as I told you, we collected data at baseline, two weeks, four weeks, six weeks, eight weeks, 12, 24 and 54 weeks. This is the data points. And if you look at it, this has to do with the fluoxetine versus CBT aspect. It’s almost mirrors… These numbers in the thing are the number of people who had suicidal behaviour. That’s really the numbers in each of these things. And you can see it doesn’t matter whether you’re on CBT or fluoxetine, it actually reduces and it seems to be better across time. But what is different is after 24 weeks, after six months, what seems to happen is the fluoxetine continues to remain low, but the CBT one goes up. And that’s a spurious effect simply because the CBT has stopped by then.
So therefore, what I’m trying to say is that this whole thing about… This is a naturalistic [inaudible 00:25:03] cohort study, it’s not a double blind controlled study, but what this shows is that in day to day practice the suicidality, whether you treat it with… when you’re depressed, whether you treated CBT or fluoxetine it seems to help, but when the treatment stops, especially the psychological interventions, it seems to again go back up, at least in this. If you look at it in all the seven cohorts again, the only one that goes up is the CBT group and everyone else is going down, again telling you that treatment is necessary longer term. You may need boosters. It’s not surprising, but, on the other hand, this is how health services currently are programmed. You give them about eight sessions, 12 sessions, and you think miraculously everything’s gone. It’s not going to. You’re going to end up with more problems afterwards if you don’t continue to boost it.
So that is all that is to do with what we found. And what I’m now going to talk about is using the data that we collected to see whether we could predict who attempted suicide versus who didn’t. And because of the data I’ve shown you, we decided that suicidal ideation should not be a priority to predict. Suicidal ideation is too variable, it is too common, and it doesn’t make much of a difference in terms of what’s happening. What is most important is suicidal behaviour. How many times have they attempted suicide and how bad is it and how lethal is it? And that’s the thing that our focus was. And so what we did was these are the four questionnaires that were there. We had data from all the [inaudible 00:26:42] raters, and what I want to do… and I will keep talking, but going back in, seven was the patients who were on either aripiprazole or risperidone for non depressed psychiatric conditions; [inaudible 00:26:57] eight is depressed in adolescence, either on CBT or fluoroxetine; [inaudible 00:27:00] nine is bronchial asthma, either on montelukast or not; and [inaudible 00:27:03] zero were the healthy controls. And that’s just the nomenclature as such and I’ll keep highlighting it as I go along. What we then did was that we developed a differentiability score, which is basically to identify those items that differentiate suicidal versus non-suicidal subjects, then calculated by computing the percentage of the given answers that the participants between the two groups and then we developed a D score. And then this is more the kind of thing that is used in the back end for machine learning. But what I’m trying to say is that it was, something that we developed with a very clear predicted strategy as opposed to just putting everything into a pot and then coming up with something.
So just to give a very idiot’s guide to what artificial intelligence machine learning is, is that if you’re looking at actual intelligence, the study of intelligent agents, basically to design an intelligent agent that perceives the same language and makes decisions, to maximise the chances of achieving its goal. That’s what it’s supposed to be. Machine learning, on the other hand, uses algorithms, learning algorithms that build a mathematical model that’s based on the data, that is the plain dataset that you give it, and in order to make predictions or decisions that can learn from the data you have and then it can explicitly be programmed to provide the task. Now, in machine learning you have three types. You have a supervised machine learning, unsupervised learning, and the third is the reinforcement learning. In the supervised learning what happens is this is for classification, and such, so this is you are telling them this is what you are going to get in your model. Now you tell us what’s the best from that. The second is the unsupervised is when you’ve dumped everything in and the computer does it and the computer spews out and says, you don’t really need to know how we came up with this decision, but this is what our algorithm is, that it is this or that.
So this is the black box part of it. And this is where the medicine as such, it is very difficult for doctors and clinicians to say suddenly we’re going to trust our judgement to a computer when we don’t even know what it used to be able to come to that conclusion. And so this bit of the machine learning is more problematic. It’s probably easier to use when you’re using it for neuroimaging or for retinal scans or things like that, but for behavioural work it’s far more difficult to do because it could be the way that it’s [inaudible 00:29:46]. Reinforcement learning is that where you end up using the reward maximisation strategy, where what you do is that you start off with the thing and then you can add and then you have an external person who is a clinician who dictates what the way it should be for what item. So it’s the human who is actually still dictating and getting it. So what we ended up doing is that we’ve dumped this because we said this is not something that we’re interested in because there’s no way I was going to want to know that the computer does something and tells me this is AOB. I don’t know how it came to that because it is too problematic an area. So we decided we would go for the supervised learning part of it.
And so what we did was we used the differentiability score and we looked at two things: accuracy is the correctly predicted label of whoever is suicidal or non-suicidal, but the total number of participants the recall is basically correctly predicted label by the total number of the label itself. In other words, this is basically the sensitivity and specificity. So if you look at this what I can share, this has to do with the neurodevelopmental disorders, without depression. And in that what you can see is that it separates out neatly into those who attempted suicide, whether… These are suicidal behaviours; this is not ideation. It is the behaviours that were calculated, and you can see that there’s a difference here. Look at these are the depressed individuals; again, you can clearly differentiate. In the mentally unwell group, that is the bronchial asthma, again clearly differentiated. And then this is the healthy population. You can actually see the only item that actually comes out of the thing is actually NSSI, just self-injury, which is without intent, which is quite… There’s an overlap between the ones who attempted versus the ones who did it.
And this is to do with when you are looking at [inaudible 00:31:49] actually just mentioned this is based on clinician ratings. When you look at this from adolescent rating, you have slightly different variables but much the same pattern. It can clearly predict who would have attempted suicide versus who wouldn’t, who did or didn’t. And this is basically… The model was to develop the model in ten percent of the data set. So only ten percent of the patients’ data was used to develop a model, then we used the next ten percent to improve the model. That means the next ten percent to test the model and see whether we needed to improve it. By the time we got to 40 percent of the dataset we had saturated the thing as such, and so therefore we then used the rest of the 60 percent of the data to test it to see how the model worked, and it did, and that’s what these data is. And this is a very simple thing. We knew the [s.l. screening 00:32:43] questionnaire, which would have said, yes or no, and what we went on was this accuracy is the same thing as specificity, and it is about 97 percent in every… It doesn’t matter whether you belong to the medical depressed [inaudible 00:32:59], and sensitivity again is about 97 percent. And it’s in the depressed group there’s a slightly different thing, but normal groups and the medically unwell group, they’re very similar and you can predict with very great accuracy whether they’re going to attempt.
And we’ve done this, repeated this, for just NSSI, without intent for to commit suicide. It’s much the same models but in different items, though, but you can do the same thing. And again, when you use the same items across all variables, all raters, whether it’s adolescents, parents or clinicians, initially you can see that you’re actually… the model remains well. So the take-home message from this was that it did not matter that you had to get multiple ratings because the model was able to predict just as well with even one person, whether it was just a parent, just a clinician, just an adolescent, you had more than 95 percent accuracy in this particular sample. And I’m not saying this is going to be replicated everywhere and I need to clearly state that but at the same time, I was so shocked that the data showed this, that we tested it from five different times, took five different methods of doing it because I couldn’t believe it. And I still… I’m hoping that someone will be able to tell us if there was something that went [inaudible 00:34:16].
So last bit of my talk is about the variables. And this is very limited because it’s… and I will show you why. So this is… We collect data from every patient coming into the clinic. This is data about a particular patient who had autonomic dysregulation, a patient most psychiatrists don’t pick up. In fact, in my clinic, the commonest reason why there’s treatment resistance, irrespective of condition… It could be for schizophrenia, it could be for depression, it could be for… is because we haven’t picked up the fundamental autonomic dysregulation when because autonomic dysregulation is not being treated… Neurologists never treat it because they think it’s not the brain, it’s just ANS, autonomic so that’s not their problem. The psychiatrists, on the other hand, they don’t really look at it because it’s too data driven and it’s too [inaudible 00:35:06], so you either end up with having waffley or woolly way of looking at things or too precise and it doesn’t really work. And so the autonomic dysregulation is something that has been present. And we picked it up because I run a 80 bed unit for acquired brain injury children and there when you have brain injury the first thing that happens is you end up having autonomic dysregulation. So that’s how it came into it, so we’ve been monitoring it and we’ve found that it exists. So what we’ve done is that we gave this particular child, just finally gave him risperone and you can see the red is the [inaudible 00:35:41] activity, the after treatment and [inaudible 00:35:45].
Now this is the same thing when it comes to heart rate. You can see this is around one hundred pre-treatment, and you can see that the majority of these are on the left, which makes it more adaptable to the child. And this is one particular case to just illustrate what can be done. You collect it longitudinally. You have three things that you collect in your heart rate variability as such. You’ve got [inaudible 00:36:07] and you’ve got [inaudible 00:36:10] activity and you use actually the [inaudible 00:36:12] data to actually calculate the artefacts as such, because if you end up running around your heart is going to go up, so it’s not going to be surprising. So the algorithm needs to have a mechanism of using data from one sensor that actually collect and answer the question in another sensor because these are all collected through different sensors. So, for example, this device collects the heart rate variability almost every 50 to 100 milliseconds. The Apple Watch, which I have, collects it every once in six minutes. And that’s the difference. So in between the six-minute data collection of heart rate variability data there’s imputation being done by a model which Apple has. And so you look at your data as if it is correctly that, but it’s actually being imputed in between and that can’t be used in cases like this.
So this is just to show you what happened. So this is [inaudible 00:37:09] activity in contact one, two, three, four. These are just chosen four patients to show you how it could be. And this is heart rate variability, and these are all the various different kinds of factors that you… You have the [inaudible 00:37:21] 50, the mean maximum of the heart rate and so on, and so you can see quickly as to why you need a full computational lab team as part of your team because I’m not going to be able to analyse all of this. So as part of my team we have an additional analyst whose job is to actually do this and investigate in real time. So what I’ve been writing here in the graph is that this is the [inaudible 00:37:47] activity on the scale of zero to five how bad it is. The green is the suicidality in terms of how bad it is, and the red is the heart rate variability and this is depression scores. So what you can see is that if you don’t look at it across time, you can end up with a false sense of having found an answer because if you just look at the data here, you might end up thinking, oh, [inaudible 00:38:15] activity is not really a problem. The suicidality and the depression is the thing, and then this comes down, the heart rate variability is starting to come down. But the implication might be completely different from that.
So the next thing to look at is this is a child who we just assessed very recently. And what you can see that this is just the first time point. And here in this child, everything’s a five. The heart rate variability is a five, most abnormal. The thing is that [s.l. EA 00:38:44] is abnormal, plus the suicidality and the depression. So what we don’t know is what’s going to happen across time here. So if we look at four… These are just four subjects and what I want to just highlight is when you’re using variables and taking technology this is being done within the context of a one-off structural assessment in clinic, so we’re not even talking about 24 hours data when they’re walking around doing everything under the sun and then you’re making inferences from that, because the variability is too high, the data storage is too high, the need to analyse is too high. This has to do with the structure and one-off session in your clinic. When you’re doing a clinic appointment, they just wear the watch and you collect the data. And even then you can see there are different patterns and that this person has increased [inaudible 00:39:29] activity and heart rate variability, and here you have a completely different pattern. So my take home message for variables is that when you’re looking at it, you have to be circumspect because you need numbers to be able to predict what kind of thing, and I just came the last bit of the last speaker’s talk, and I think when it comes to all those different motivating factors which are being in the model, they may have very different perspectives as such.
So to conclude, what we have managed to achieve is we’ve looked at all of the data available, we’ve developed instruments, we’ve actually got it on [inaudible 00:40:12] online, we’ve actually validated all of them; they’re all published now. And then we’ve done the machine learning strategies to be able to try and identify the prediction modelling. And we’ve got scales just based on 20 items in the end, which takes a patient up to five minutes to eight minutes to complete. It can be done on the phone; it can be done on anything. That’s the product in the end. Now, that has to be tested in the real world in patients as such to see what it does. And my take on the variables part of it is that I think it’s likely that you will need a hell of a lot more than we can actually provide in effect because variability is too high and you may need to find a subgroup of individuals with [inaudible 00:40:55] activity and then focus on that subgroup to see what’s common to them. And that’s the pattern, I think, which will help in the long term. So thank you.